Enhancing Knowledge Management with Generative AI
Introduction
In the fast-paced and dynamic information landscape, businesses and organizations face a constant challenge in managing and harnessing knowledge effectively. As the volume of data continues to grow exponentially, traditional knowledge management approaches often fall short of providing timely and relevant insights. Enter Generative AI – a transformative technology that has the potential to revolutionize knowledge management.
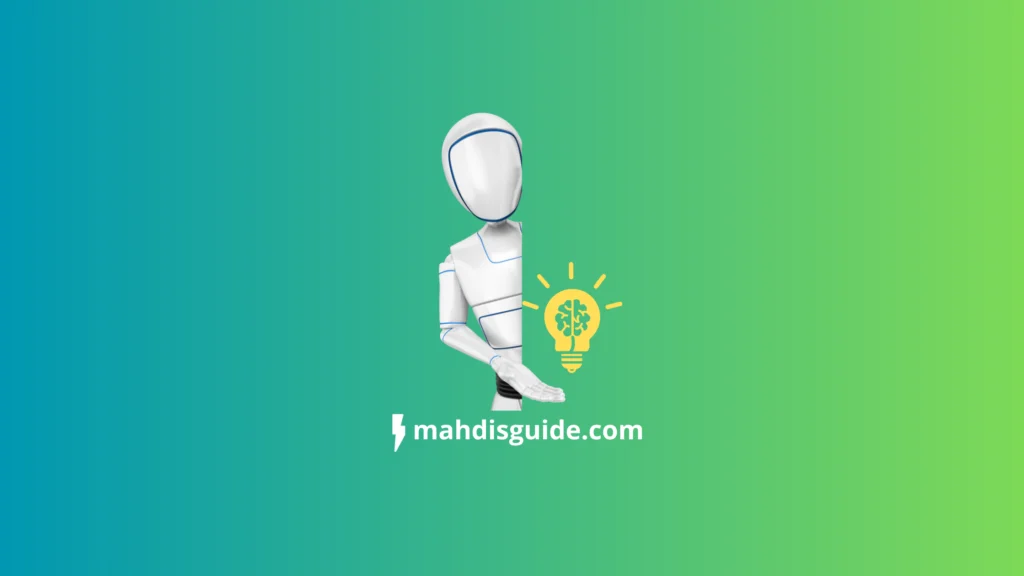
Understanding Generative AI
Generative Artificial Intelligence (Generative AI) refers to a class of AI models designed to generate new content, whether it be text, images, or other forms of data. Unlike traditional AI systems that operate based on pre-programmed rules, generative models learn from vast datasets and are capable of producing creative and contextually relevant outputs.
Bridging the Gap: Generative AI and Knowledge Management Processes
1. Content Creation and Summarization
One of the primary ways generative AI can enhance knowledge management practices is through its ability to create and summarize content. These models can generate reports, articles, and summaries with remarkable speed and accuracy, automating the labor-intensive process of content creation. This not only saves time but also ensures that knowledge organization is disseminated coherently and consistently.
2. Data Extraction and Categorization
Generative AI capabilities excel at extracting valuable information from unstructured data. It can sift through vast amounts of text, identifying key concepts and patterns and categorizing information accordingly. This capability is particularly beneficial for knowledge management systems that rely on structured data for effective organizational knowledge and retrieval.
3. Personalized Knowledge Delivery
Tailoring information delivery to individual preferences is a key aspect of effective knowledge management. Generative AI can analyze user behavior and preferences to create personalized content recommendations. This ensures that users receive information that is not only relevant to their roles but also aligns with their specific interests and needs.
Overcoming Challenges: Generative AI and Knowledge Quality
1. Content Enhancement and Correction
Generative AI models can play a pivotal role in enhancing the quality of existing knowledge repositories. By analyzing and understanding the context, these models can identify inaccuracies, outdated information, or knowledge gap. Subsequently, they can suggest corrections or updates, ensuring the knowledge base remains accurate with up-to-date information.
2. Semantic Understanding for Better Context
Understanding context is crucial in knowledge management, and generative AI models excel in semantic understanding. They can grasp the nuances of language, discerning the meaning behind words and phrases. This capability enables these models to provide more contextually relevant information, reducing the likelihood of misinterpretation.
The Evolution of Knowledge Search: NLP and Generative AI
1. Natural Language Processing (NLP) Integration
Generative AI models, particularly those built on natural language processing (NLP) architectures, can significantly enhance the search capabilities of knowledge management systems. Users can interact with these systems using natural language queries, and the AI model can interpret and generate responses in a way that closely mirrors human language.
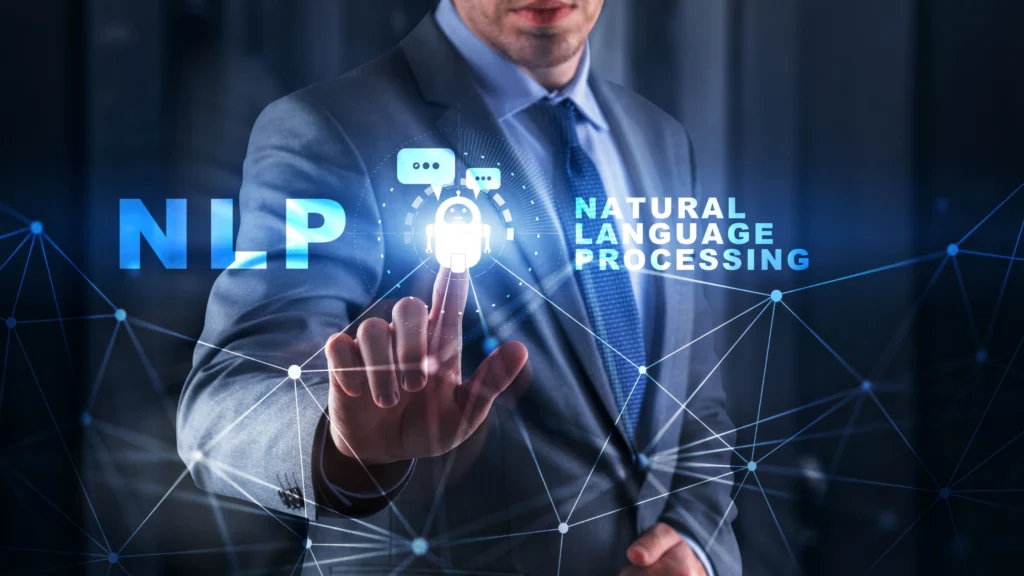
2. Conversational Knowledge Interfaces
Taking NLP a step further, generative AI tools can facilitate the development of conversational knowledge interfaces. Users can engage in dialogues with these interfaces, asking questions and receiving detailed, contextually rich responses. This improves the user experience and fosters a more intuitive and interactive approach to accessing information.
Implementation Challenges and Considerations
1. Data Privacy and Security
As generative AI relies on vast datasets to learn and generate content, concerns about data privacy and security become paramount. Organizations must implement robust measures to ensure that sensitive information is protected and that the use of generative AI aligns with relevant regulations and standards.
2. Ethical Considerations in Content Generation
The ability of generative AI to create content raises ethical concerns, particularly in instances where misinformation or biased content may be generated. Organizations must establish ethical guidelines and frameworks to govern the use of generative AI in knowledge management, promoting responsible and unbiased content creation.
The Road Ahead for Generative AI in Knowledge Management
1. Continuous Learning and Adaptation
The field of generative AI is evolving rapidly, and future models are likely to exhibit even more advanced capabilities. Continuous learning and adaptation will be key trends, enabling AI systems to stay abreast of the latest developments and dynamically adjust their knowledge management strategies.
2. Human-AI Collaboration in Knowledge Curation
While generative AI can automate many knowledge management tasks, the human touch remains invaluable. Future trends may see an increased emphasis on collaboration between humans and AI, with AI systems supporting human experts in curating and refining knowledge repositories.
Conclusion
Generative AI represents a paradigm shift in how organizations approach knowledge management. By harnessing the power of these advanced models, businesses can not only streamline knowledge creation and dissemination but also enhance the quality and accessibility of their information. As the technology continues to mature, the synergy between generative AI and knowledge management holds the promise of a more intelligent, efficient, and adaptive approach to handling the vast seas of information in the digital age. You can have a look at our website for more useful content.